Discover the concept of confidential computing and its crucial role in enhancing data privacy in today’s digital landscape.
Rishabh, what inspired you to co-found Opaque Systems, and how does your background in computer science and cryptography contribute to the company’s success?
The founding of Opaque Systems goes back to the RISELab at UC Berkeley. While at UC Berkeley, I and a team of world-renowned security and systems researchers and practitioners – including Raluca Ada Popa (UC Berkeley professor and co-founder of Opaque Systems), Ion Stoica (co-founder of Databricks), and industry visionaries, Wenting Zheng, and Chester Leung — developed MC2, an open source project focused on enabling multi-party analytics and AI on confidential data. The massive enterprise demand for these capabilities led to five years of extensive research that became the basis for the Opaque Systems you see today.
While receiving my Ph.D. in computer science, I spent years researching confidential computing and the seemingly untouchable challenge of making collaboration on encrypted data possible. I saw the market need and dedicated myself to developing a platform where multiple organizations could securely collaborate on data throughout its entire lifecycle and unlock aspects of data that had previously been hidden from organizations. In addition to my background and my co-founders’, we received early access to Intel’s SGX hardware enclaves for confidential computing — years before it became commercially available — which gave us a head start on innovation and research.
Can you tell us more about Opaque Systems and its mission to provide a confidential computing platform for collaborative analytics, AI, and data sharing at scale?
Our mission is to help organizations protect their sensitive data and realize the massive opportunity to leverage this untapped treasure trove of data. Much of what we’ve been doing since the launch of Opaque’s Confidential Computing Platform in December of 2022, has been focused on enabling use cases for secure data sharing, multi-party analytics and Data Clean Rooms (DCRs) for financial services organization, in AdTech, healthcare and more.
At the recent Confidential Computing Summit we also demonstrated the role of confidential computing for privacy-preserving LLM’s, with the recent AI advancements. Our latest innovations to the Opaque Confidential Computing platform are focused on protecting the confidentiality of data used in LLMs (confidential AI) and zero-trust DCRs optimized for Microsoft Azure Confidential Computing to enable secure multi-party collaboration on encrypted/confidential data. These innovations allow organizations to quickly and securely use LLMs to analyze confidential data without sharing or revealing the underlying raw data — keeping data encrypted and secure throughout its lifecycle. It is an important step towards a future where confidential computing becomes the standard for computing, and we’re really excited about its potential.
Could you explain the concept of confidential computing and its significance in today’s data privacy landscape?
With data privacy regulations becoming more stringent, securing confidential and sensitive data is an urgent priority for organizations globally. Confidential computing — projected to be a $54B market by 2026 by the Everest Group — provides a solution using TEEs or ‘enclaves’ that encrypt data during computation, isolating it from access, exposure, and threats. When used to its full potential, confidential computing can help solve today’s biggest data privacy concerns by providing a secure data processing environment and ensuring organizations comply with new privacy regulations and protect against growing attack surfaces.
We’ve taken that further with the Opaque Confidential Computing Platform by enabling secure multi-party analytics and AI in these enclaves. Through our platform, financial institutions, for example, can be confident that their data remains confidential even when pooling data sets together from different business units/organizations to fight financial crime better and prevent money laundering — as just one example use case.
The Confidential Computing Consortium and Opaque Systems recently co-hosted the inaugural Confidential Computing Summit. What were the main objectives of this summit, and what were the highlights?
The inaugural Confidential Computing Summit earlier this summer addressed the state of the industry and highlighted innovations and breakthrough use cases involving confidential data. Our main objective was to showcase confidential computing’s potential and educate the industry on its robust use cases. The Summit aimed to bring together the premier technology providers, researchers, as well as existing users to help organizations advance confidential computing. While we had some of the biggest names in confidential computing innovation speak, some highlights included our panels on confidential computing’s trending applications and use cases, the surging demand for DCRs, and the state of Generative AI security and privacy.
The Opaque Systems team also launched innovations to our Confidential Computing Platform, enabling confidential AI and zero-trust DCR capabilities. Through privacy-preserving generative AI, our new confidential AI functionality enables an enterprise to use LLMs on sensitive data while still preserving its confidentiality — one of the biggest issues in today’s business world as more organizations use LLMs like GPT-4. Our zero-trust DCRs, on the other hand, are geared towards enabling multi-party collaboration on confidential data to unlock its business value without sharing or revealing the underlying raw data. This is particularly applicable to the AdTech industry, where collaboration on sensitive datasets can unlock net new use cases and opportunities for clients.
With data privacy regulations becoming more stringent, how does Confidential Computing address the urgent need to secure confidential and sensitive data for organizations globally?
As organizations look to enhance data privacy and adhere to more stringent regulations, they must go beyond data encryption at rest or in transit alone. It’s imperative to adopt solutions such as confidential computing that keep data encrypted/protected when in use — in memory and during computation — to prevent the exposure of confidential data to unauthorized parties.
Data security is at greater risk, especially with the rise in generative AI models and LLMs. The big privacy problem with LLMs is that unwanted parties (such as the service provider) can gain access to sensitive information such as proprietary code, confidential customer data, or other forms of IP if they’re not secured and encrypted during model processing. Organizations with proprietary models that are running LLMs can also run into IP issues in some cases, if they need to deploy their proprietary model within the customer organization — opening the doors to increased data exposure and risk.
Confidential computing allows organizations to use groundbreaking confidential AI capabilities to solve data privacy concerns. Opaque’s platform, for instance, provides a safeguard against the exposure of confidential data to unauthorized parties through multiple layers of protection for sensitive data against potential cyber-attacks or data breaches through a powerful combination of secure hardware enclaves and cryptographic fortification.
The Everest Group forecasts that the Confidential Computing market will reach a $54B market opportunity by 2026. How do you see this market evolving, and what factors will contribute to its growth?
Over the last few years, organizations have realized the benefits of sharing data across teams and organizations. With the growing amount of sensitive data rising within businesses daily, the need for confidential computing has never been greater. One of the biggest reasons confidential computing rose to the top of the market was the initial support from all major cloud vendors, including Microsoft Azure, Google Cloud, and Amazon Web Services, and major chip manufacturers, such as Intel and AMD. Now, in the era of generative AI, we’ll see confidential computing continue to grow and become a must-add tool for all industries looking to collaborate on sensitive information.
Who attended the Confidential Computing Summit? What were the key topics discussed, and how did they relate to secure data sharing, data processing, multi-party analytics and AI, privacy-enhancing technologies, and confidential computing cloud platforms?
The majority of the attendees were technical experts and those whose roles fell into the categories of innovator, executive, regulator, business leader, security expert, data scientist, data analyst, AI/ machine learning practitioner, data privacy expert, or researcher.
Attendees heard from experts from Google, Microsoft Azure Confidential Computing, VMware, Accenture, PwC and Ernst and Young, among others, and left with a greater knowledge of confidential computing, its emergence as a key solution for the future of data security and privacy, and its many powerful use cases across industries, including but not limited to financial services, insurance, healthcare, manufacturing, AdTech, and Web3.
As a co-founder of Opaque Systems, what were your expectations for the Confidential Computing Summit, and how do you envision the summit contributing to the adoption and advancement of confidential computing?
We had really high hopes for the Confidential Computing Summit, and I’m happy to say we exceeded those expectations and had an exceptional turnout in San Francisco. Opaque Systems and the Confidential Computing Consortium (CCC) saw the summit as a way to showcase confidential computing’s potential and educate attendees on its powerful use cases and shed light on some of the creative innovations happening in academia, as well as at some of the top companies in the market. We believe that greater visibility and more understanding of confidential computing would, in turn, lead to greater industry awareness and understanding that this is the key to addressing ongoing data privacy and regulation concerns.
Opaque Systems commercializes the open-source MC2 technology invented at UC Berkeley. Could you explain how this technology enables secure data sharing and analysis among multiple parties while maintaining confidentiality?
MC2 (Multi-Party Collaboration and Coopetition) enables rich analytics and machine learning on encrypted data, ensuring that data remains concealed even when processed. Through a temporary “black box” method via secure enclaves, the data being used remains confidential to the server running the job. It may sound contradictory, but it’s true: multiple data owners can jointly run analytics or run ML models on their collective data without revealing that data to anyone else. This alleviates concerns about offloading confidential workloads to untrusted third parties or cloud providers. MC2 solves the tension between expanding cloud adoption, the need for data sharing, and the increasing concern over data privacy.
Can you elaborate on the secure hardware enclaves and cryptographic fortification utilized by the Opaque Platform to ensure secure, fast, and scalable computations?
Opaque uses confidential computing technology to leverage specialized hardware. Our privacy-enhancing technology ensures that datasets are encrypted throughout the entire analysis process — allowing multiple parties to collaborate on analytics and AI use cases without compromising the confidentiality of the individual data. The data remains encrypted and protected during its entire lifecycle. The specific innovation breakthroughs for our platform include:
- High-performance analytics and AI/ML on encrypted data using familiar tools. The ability to isolate sensitive data in TEEs, including enclaves and confidential VMs, and perform collaborative, scalable analytics and machine learning directly on encrypted data using familiar tools such as Apache Spark and notebooks.
- Inter- and Intra-company collaborative analytics, AI/ML, and data sharing. Multiple data teams within and across organizations can collaborate to securely share encrypted data, perform collaborative analytics and ML on data while encrypted and enforce data and computation policies. Each party is privy only to their specific data and insights.
- Multidimensional scaling across enclaves, data sources, and multiple parties. Provides a simplified data management approach with secure access across enclave clusters and the ability to automate cluster orchestration, monitoring, and management across multiple workspaces without operational disruption.
How does the Opaque Platform address the challenges of maintaining data confidentiality and protecting data end-to-end, especially in industries such as financial services, insurance, healthcare, manufacturing, adtech, and web3?
Our technology allows multiple organizations and data owners to collaborate securely while remaining in regulatory compliance. Our Confidential Computing Platform opens up significant opportunities for collaboration where various barriers previously existed for value extraction. For example, financial institutions can now collaborate on sensitive data for regulatory and commercial reasons — without exposing the data itself — and analyze joint data to identify and prevent fraud, and deepen their insights into money laundering activity and risk measurement. In healthcare, we’re seeing use cases around collaborating on sensitive data for faster drug discovery, patient profiling, and better insights into quality of care.
As I mentioned earlier, our new data clean room innovations lean heavily into Adtech, as it’s a primary use case for confidential computing and DCRs. Opaque’s AI-driven Platform functions as a secure data room and empowers AdTech companies to unlock the value of confidential and sensitive data by enabling useful analytics and machine learning on fully encrypted data at scale. This ensures encryption not just at rest and in transit but also in use — allowing users to share insights from valuable data without compromising the privacy of their first-party data.
In your opinion, what is the future of confidential computing, and how do you see Opaque Systems contributing to its development and adoption?
Confidential computing is the future of data collaboration and analysis. Without a secure platform that protects data privacy from end-to-end, organizations will be pigeon-toed into silos and unable to innovate to their full potential.
This is becoming particularly necessary for preserving the security and privacy of data used in AI models and LLMs. With Opaque, our Confidential Computing Platform allows customers to encrypt their prompts and sensitive data to derive the benefit of the generative AI without exposing private and proprietary information. This is done alongside policy control, auditing transparency, and full data custody. With broader support for confidential AI use cases, the Opaque platform will also provide safeguards for machine learning and AI models to execute encrypted data inside Trusted Executions Environments (TEEs), preventing exposure to unauthorized parties.
Are there any partnerships or collaborations that Opaque Systems has formed to enhance its confidential computing capabilities or expand its reach?
We just recently announced a strategic partnership with Roqad, an identity solutions provider, to enable seamless multi-party data collaboration through data clean rooms (DCRs) while still adhering to evolving privacy rules and regulations. We also closely collaborate with leading global professional services firms as well as tech giants such as Intel and Microsoft Azure; Accenture co-presented with us at the Confidential Computing Summit regarding our Data Clean Room solution.
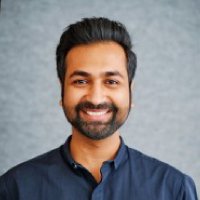
Rishabh Poddar
CEO and Co-founder of Opaque Systems
Dr. Rishabh Poddar is the CEO and Co-Founder of Opaque Systems, a confidential computing platform for collaborative analytics, AI, and data sharing at scale. Rishabh holds several US patents and has authored over 20 research papers in computer security, systems, and applied cryptography. Rishabh received his Ph.D. and MS degrees in Computer Science from UC Berkeley and an undergraduate degree in Computer Science from the Indian Institute of Technology (IIT) Kharagpur. Previously, Rishabh was a researcher at IBM Research and a management consultant at The Boston Consulting Group.